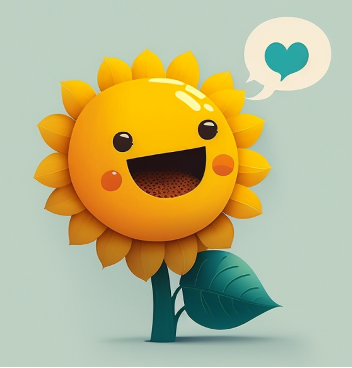
[1] The image is created with Midjourney
Highlights
- SambaNova, in collaboration with Together, is excited to present BLOOMChat, a 176 billion parameter multilingual chat large language model (LLM). BLOOMChat is available for research and commercial use cases under a modified version of Apache 2.0, which includes RAIL’s use-based restrictions passed down from BLOOM.
- BLOOMChat is a new, open, multilingual chat LLM that:
- Is trained on SambaNova RDUs (Reconfigurable Dataflow Units)
- Achieves a win-rate of 45.25% compared to GPT-4‘s 54.75% across 6 languages in a human preference study.
- Is preferred 66% of the time compared to mainstream open-source chat LLMs across 6 languages in a human preference study.
- Shows strong performance on WMT translation tasks by leading the results among BLOOM variants and mainstream open-source chat models.
- Inspired by prior work that instruction tuning in one language can benefit performance in another language in multilingual models, we instruction-tuned BLOOM (176B) with English-focused assistant-style conversation datasets including OIG from OpenChatKit , Dolly 2.0, and OASST1 datasets. Despite only fine-tuning in English, we observed substantial increases in chat quality in non-English languages.
- While this is still an early study, we hope BLOOMChat serves as a valuable resource for the open-source community and as a stepping stone towards further advancements in the field.
- BLOOMChat is available now [Chat with me!] for a limited time for live chatting on HuggingFace (model hosting and frontend UI provided by Together).
Introduction
Proprietary large language models (LLMs) like GPT-4 and PaLM 2 have significantly improved multilingual chat capability compared to their predecessors, ushering in a new age of multilingual language understanding and interaction. These advancements, however, have been largely confined to proprietary models.
Meanwhile open-source LLMs are gaining in popularity due to their ability to democratize LLM access, offer transparency, reduce ownership costs, and allow for customization. Notably, in contrast to using closed API services, leveraging open-source models mitigates privacy concerns and effectively sidesteps the risks tied to sensitive data leakage.
The open-source community has recently made significant progress for chat-based LLMs in English, yet multilingual chat capability remains largely untapped. Recent releases such as OpenChatKit, Dolly 2.0, OASST1, and models like OpenAssistant-30B, LLaMA-Adapter-V2-65B and Vicuna-13b have considerably narrowed the gap with closed models. However, the focus has been mainly on English-centric models[1], leaving the potential of open-source LLMs in delivering comparable multilingual chat capability largely unexplored. More than 80% of the world’s population are non-English speakers[2], so multilingual chat models are essential to democratizing access to AI and expanding the research community that can participate in and drive AI innovation.
To address this gap, SambaNova and Together trained BLOOMChat on SambaNova DataScale systems using SambaNova’s unique Reconfigurable Dataflow Architecture. BLOOMChat is a 176B multilingual chat LLM built on top of BLOOM from the BigScience organization, and fine-tuned on OIG from OpenChatKit, Dolly 2.0, and OASST1. BLOOM is already the largest multilingual open model, trained on 46 languages and developed by an international collaboration of more than 1000 researchers. By fine-tuning it on open conversation and alignment datasets, we take these core capabilities and extend them into the chat arena. To increase accessibility and to support the open-source community, SambaNova is releasing BLOOMChat under a modified version of the Apache 2.0 license, which includes use-based restrictions from BLOOM’s RAIL license.
Sure, here’s an example of a survey that could be used to gather information on people’s eating and cooking habits:
- Introduction: In this section, you would provide some context for the survey by explaining why you’re conducting it and what your goals are.
- Demographic questions: These questions would help you understand who is responding to the survey and how they might differ in their eating and cooking habits based on factors such as age, gender, ethnicity, education level, income, etc.
- Eating habits questions: Here, you’d ask questions related to how often people eat out or cook at home, what types of foods they’re interested in trying new things with (e.g., ethnic cuisine), whether they have any dietary restrictions or preferences, how much time they typically spend preparing meals each week, etc.
- Cooking habits questions: This section would focus more specifically on how people prepare food when they do cook at home. You’d want to know if they use recipes, follow online tutorials, improvise most of the time, prefer pre-made ingredients, etc.
- Future interests: Finally, you’d like to know if respondents are interested in learning more about nutrition/food safety, meal planning, budget-friendly recipes, etc. This will allow you to tailor future surveys and/or content creation efforts towards areas where there seems to be demand from your audience.
Overall, this type of survey can give you valuable insights into your target audience’s eating and cooking habits which can inform decisions around product development, marketing strategies, recipe development, etc.
请向我推荐一部周末看的电影并说明原因。
(Recommend a movie for me to watch during the weekend and explain the reason.)
强烈向您推荐《美丽人生》这部影片。该片由罗伯托·贝尼尼自导自演,讲述了二战时期一对犹太父子被送进了纳粹集中营,父亲用自己的想象力为儿子编织了一个美好童真的世界,让儿子的心灵没有受到丝毫伤害的故事。
该片被誉为“最伟大的战争片”、“人类有史以来最佳影片之一”,豆瓣评分高达9.6分。它不仅是一部感人的亲情片,更是一部富含哲理的励志片和人生启示录。片中父亲的乐观豁达、幽默风趣的人生态度,对任何事都抱有积极热情的态度,即使在残酷无比的集中营里也是如此,深深地感染着观众。同时,这也是一部关于爱与希望的电影,让我们更加珍惜当下所拥有的一切,学会如何去爱身边的每一个人。
总之,这是一部值得一看再看的好电影,相信您一定会喜欢的!
दिल्ली से जयपुर कैसे जाये? बस से या कार से? दोनो के बारे मे विस्तार मे बताये.
(How do I go from Delhi to Jaipur? Bus or car? Details please.)
दिल्ली से जयपुर जाने का सबसे आसान और तेज़ तरीका है कि आप अपनी खुद की कार लेकर जाएं, क्योंकि यह आपको अपने समय पर निर्भर रहने की स्वतंत्रता देता है। हालांकि, यदि आपके पास अपनी कार नहीं है, तो आप दिल्ली से जयपुर तक एक निजी वाहन किराए पर ले सकते हैं। यदि आप सार्वजनिक परिवहन (बस) का उपयोग करना चाहते हैं, तो दिल्ली में कई सरकारी और निजी बस सेवाएं उपलब्ध हैं जो जयपुर को जोड़ती हैं। दिल्ली से जयपुर जाने वाली कुछ प्रमुख बसें हैं-
- राजस्थान रोडवेज: ये राज्य सरकार द्वारा संचालित बसें हैं जो दिल्ली से जयपुर के बीच चलती हैं। ये नियमित रूप से चलती हैं और इनके लिए अग्रिम आरक्षण आवश्यक नहीं है।
- ALSA: यह एक निजी कंपनी है जो दिल्ली से जयपुर के बीच नियमित रूप से चलती है। इसके टिकटों की बुकिंग ऑनलाइन और ऑफलाइन दोनों माध्यमों से की जा सकती है।
- RedBus: यह एक ऑनलाइन बस बुकिंग वेबसाइट है जहां से आप दिल्ली से जयपुर के बीच चलनेवाली विभिन्न कंपनियों की बसों में सीट आरक्षित करा सकते हैं।
- Ola and Uber: इन ऐप्स का उपयोग करके आप दिल्ली से जयपुर तक कैब राइड बुक कर सकते हैं, लेकिन ध्यान रखें कि यह विकल्प सस्ता नहीं होगा। दिल्ली से जयपुर जाने का सबसे अच्छा विकल्प आपकी व्यक्तिगत आवश्यकताओं, बजट, और उपलब्धता पर निर्भर करता है। यदि आप अपनी खुद की कार रखते हैं, तो इसका लाभ उठाएं अन्यथा किसी भी सार्वजनिक परिवहन साधन का चयन करें जो आपकी आवश्यकताओं को पूरा करे।
¿Me puedes dar algunas recomendaciones de juegos? Estoy buscando juegos de rol con un sistema de magia único, un sistema de construcción único y una buena historia. Por favor limítate a juegos que se puedan jugar en PC directamente, o por emulación.
(Can you provide some game recommendations, I’m looking for a Role Playing Game with a unique magic system, an in depth crafting system and a good story. Please mainly limit the games to ones that can be played on PC either directly or through)
Claro! Aquí te dejo tres recomendaciones:
- The Outer Worlds: es un juego de rol de ciencia ficción con un sistema de magia basado en la tecnología alienígena que permite al jugador personalizar sus habilidades para adaptarse a su estilo de juego.
- Divinity: Original Sin 2 – Definitive Edition: este juego combina elementos de RPG con estrategia en tiempo real (RTS), lo que le da al jugador un control total sobre cómo construir y mejorar su personaje y el mundo que lo rodea.
- Pillars of Eternity: esta aventura de fantasía oscura tiene lugar en un vasto mundo abierto donde los jugadores pueden elegir entre diferentes clases y desarrollar relaciones con otros personajes del juego. La mecánica de combate está basada en turnos y utiliza hechizos y armas cuerpo a cuerpo.
Estos son solo algunos ejemplos de juegos que podrían interesarte según tus criterios. Hay muchos más títulos disponibles en el mercado que podrían ajustarse a tu gusto, así que no dudes en explorar otras opciones si estos juegos no cumplen con tus expectativas.
Je me suis séparée de ma copine et je suis très triste. Est ce que tu peux me donner des conseils?
(I broke up with my girlfriend and I am feeling so sad. Can you give me some suggestions?)
Bien sûr, voici quelques conseils pour vous aider à traverser cette période difficile :
- Parlez avec vos amis ou votre famille : Partager vos sentiments peut vous aider à vous sentir mieux.
- Faites quelque chose qui vous rend heureux : Pratiquer une activité que vous aimez, comme le sport, la lecture ou les passe-temps créatifs, peut vous aider à oublier vos soucis pendant un moment.
- Pratiquez la pleine conscience : La méditation de pleine conscience peut vous aider à réduire le stress et l’anxiété.
- Trouvez du réconfort dans votre routine quotidienne : Continuez à faire les choses que vous faites habituellement, telles que manger sainement et dormir suffisamment, cela peut vous aider à vous sentir plus en contrôle.
- Ne restez pas seul : Essayez de sortir et de voir des gens, même si c’est juste pour prendre un café ou aller au cinéma.
- Soyez patient : Le temps guérit toutes les blessures, alors donnez-vous le temps de guérir.
هل العمل نصف دوام فكرة جيدة لمراهقين بين عمر ١٥ و ١٩ عام
(Is working part-time a good idea for teenagers between 15 and 19 years old?)
نعم، يمكن أن تكون فكرة جيدة لبعض المراهقين في هذا العمر. هناك العديد من الفوائد التي قد يحققها المراهقون إذا عملوا بنصف دوام:
-يساعدهم على تعلم المسؤولية وإدارة الوقت: عندما يعمل المراهق بنصف دوام، فإنه يتعلم كيفية إدارة وقته بشكل فعال وكيفية تقسيم ساعات عمله إلى مهام أصغر.
– يحسن مهارات التواصل والعمل الجماعي: غالبًا ما يكون مكان العمل بيئة متعددة الثقافات حيث يلتقي المراهقون بمجموعة متنوعة من الأشخاص ويتعلمون كيفية التعاون معهم وتبادل الأفكار.
– يوفر فرصة للتعرف على عالم العمل: يتيح العمل للمراهق التعرف على عالم العمل واكتساب خبرة عملية قيمة ستساعده لاحقًا عند البحث عن وظيفة أو بدء حياته المهنية.
-يعزز الشعور بالمسؤولية والثقة بالنفس: عندما يعمل المراهق بنصف دوام ويحصل على دخل خاص به، فإنه يكتسب شعورًا أكبر بالمسؤولية والثقة بالنفس.
ومع ذلك، لا ينبغي إجبار المراهقين على العمل بنصف دوام دون موافقتهم المسبقة. يجب أيضًا مراعاة أعمارهم وقدراتهم البدنية والعقلية قبل توظيفهم.
BLOOMChat chat example in 6 different languages
Prior work has shown how finetuning large multilingual language models on English tasks with English prompts allows for task generalization to non-English languages that appear in the pre-training corpus of the model. We build on top of this premise to strengthen the multilingual chat capability of the model by aligning on rich English-focused assistant-style conversational datasets. The results of this training showcased significantly improved chat experiences across multiple languages on which the original model was pre-trained on, but absent from the alignment corpus.
When compared to GPT-4, BLOOMChat responses were preferred 45.25% of times during human evaluations across 6 languages. When compared against 4 other open-source chat-aligned models in the same 6 languages, BLOOMChat’s responses were ranked as the best 65.92% of the time, effectively filling the gap in multilingual chat capability in the open-source field. In our preliminary study using BLOOMChat for cross-lingual NLP tasks, we found BLOOMChat outperformed other BLOOM variants and mainstream open-source chat models in the WMT translation benchmark. We do want to note that some of these models that we compared with are not adapted for multilingual settings. But the absence of an alternative in the open source community led to the current comparisons.
Our findings suggest that with the right techniques, it is possible to build on top of open-source LLMs to achieve strong multilingual chat capability. We hope that our research findings, and the release of the BLOOMChat checkpoint, can contribute to the ongoing discourse in the open-source community and inspire further advancements in the field of LLMs.
[2] OpenAssistant/oasst1 contains 57.2% non-english chat data, but the OpenAssistant models are all instruction tuned from english-pre-trained model such as Pythia and Llama
[3] https://www.statista.com/statistics/266808/the-most-spoken-languages-worldwide/
Method
Data Collection
In developing BLOOMChat, we aimed to combine the benefits of synthetic conversation data and high-quality human-written examples. We first utilized OpenChatKit, a large synthetic conversation dataset, to lay the foundation for the model’s chat capability. To enhance the model’s performance and human-alignment, we then trained it on human-generated datasets Dolly 2.0 and OASST1, which contain small amounts of higher-quality examples.
Training
We trained BLOOMChat on SambaNova DataScale systems with RDUs (Reconfigurable Dataflow Units). We started with BLOOM-176B, an open-source multilingual 176B GPT model pre-trained by the BigScience group. We then instruction-tuned BLOOM-176B on OpenChatKit with each data source subsampled to 100k for one epoch, followed by three epochs over the combined OpenChatKit and Dolly-v2.
All of the code used to prepare the datasets and the scripts to run training and inference are open-sourced and freely available in SambaNova’s GitHub.
Results
We use qualitative and quantitative measures to evaluate BLOOMChat’s multilingual chat capability as well as cross-lingual task capability. We conducted two human evaluation experiments, Human Preference Rankings and Model Quality Ratings to measure response quality. We also benchmarked the model on WMT translation as a preliminary study of its ability on cross lingual tasks.
Experiment 1: Human Preference Rankings
We ran two experiments with volunteers from SambaNova to evaluate BLOOMChat against baseline chat models: human preference rankings and model quality ratings. The volunteers had no knowledge of the source model when providing rankings or ratings. These experiments were run in English and five additional languages: Arabic, Chinese, French, Hindi, and Spanish. These languages are present in BLOOM‘s pre-training corpus and also represent the most spoken languages 2023. All participants were asked to complete surveys in languages where they had native-level proficiency.
For the human preference rankings experiment, we wanted to compare the chat capability of our model in multiple languages with existing open-source models, as well as select closed source models. We use the 22 prompts presented in Appendix E of “OpenAssistant Conversations” for English evaluation. Volunteers from SambaNova manually translated the prompts into their respective native languages. Another set of volunteers verified the translations to be semantically and syntactically correct.
Volunteers ranked responses from each model according to their personal preferences. At least four volunteers submitted rankings for each language. As before, the volunteers had no knowledge of the source model when ranking responses. We first compare BLOOMChat with leading open-source models and then compare with GPT-4.
Comparison with open-source models
We compare BLOOMChat against the following models:
- OpenAssistant-30B: an open-source state-of-the-art chat-aligned LLM
- LLaMA-Adapter-V2-65B: an open-source state-of-the-art chat-aligned LLM
- BLOOMZ (176B): an open-source LLM instruction tuned from BLOOM (176B)
Figure 1: BLOOMChat vs Baseline Models in Human Preference Ranking
With a total of 1158 comparisons submitted by 51 volunteers across all models and 6 languages, we found that BLOOMChat responses were preferred in 65.92% of the submissions. This suggests that BLOOMChat has shown promising multilingual chat capability compared to other similar open-source models. For a more comprehensive breakdown of the results per language, please refer to Appendix B.1.
Comparison with closed model: GPT-4
Figure 2: BLOOMChat vs GPT-4 in Human Preference Ranking
With 1072 comparisons submitted by 45 volunteers in 6 languages, we find that BLOOMChat achieves competitive results against GPT-4, with a 44.97% win-rate over OpenAI’s model. The result demonstrates that BLOOMChat has made promising progress in bridging the gap between open-source and closed models in multilingual chat capability. Please see Appendix B.1 for a detailed breakdown of winning votes by language.
Experiment 2: Model Quality Ratings
In this experiment, we wanted to verify the quality of BLOOMChat’s generated text in multiple languages. To that end, we follow the procedures outlined in Section 5.4 of the Self-Instruct paper. To effectively gauge our model’s multilingual abilities, we filtered out unqualified prompts for chat or translation and curated 32 prompts from the evaluation prompt pool from the Self-Instruct paper. The full procedure for prompt selection and our exhaustive list of prompts can be found in Appendix C. As with Experiment 1, volunteers from SambaNova manually translated each prompt into their respective native languages. At least one other volunteer verified each translation or offered an alternative, equivalent translation. Translations were not finalized until each prompt had at least two native speakers agree on the translation(s). In case of multiple equivalent translations, one was randomly selected for the final dataset.
Figure 3: BLOOMChat Human Quality Ratings in 6 Languages
For each response generated by BLOOMChat, volunteers gave one of four quality ratings (the same as those used by the authors of Self-Instruct). In total, 1408 comparisons were submitted by 44 human volunteers across 6 different languages. A detailed breakdown of votes per language can be found in Appendix D.
We found that 81.8% of responses were classified as “Correct” or “Acceptable with Minor Imperfections”. And notably, BLOOMChat achieved “Correct” or “Acceptable” ratings of over 70% in every language, despite its primary training on English chat alignment data.
We release our translated prompts for both experiments as HuggingFace datasets in hopes of bolstering further research into multilingual chat.
- Experiment 1 prompts: https://huggingface.co/datasets/sambanovasystems/xOA22
- Experiment 2 prompts: https://huggingface.co/datasets/sambanovasystems/x-self-instruct-seed-32
Experiment 3: WMT translation task
To get a preliminary understanding of the capability of the model in solving cross-lingual NLP tasks, we evaluated the model’s translation capability on the WMT translation task. We evaluated all models on WMT14-fr-en, WMT14-hi-en since they were reported as baseline in BLOOM paper. Also, we added WMT18-zh-en to cover another major language.
We chose the best known multilingual models, BLOOM variants and GPT-4, as our baselines. It’s important to note that the open-source chat models are pre-trained with an English corpus, thus a lack of comprehensive translation capability is expected. Therefore, the figures we’re presenting for these models are not meant for direct comparison but rather to give an understanding of the current state of the field.
BLOOMChat demonstrates better multilinguality compared to its variants BLOOM and BLOOMZ. It boosted the average BLEU score for BLOOM by 89.4%[1] and BLOOMZ by 86.4%. We evaluated all models using the bigscience lm-eval-harness repo with the version-target style prompt for every model with their corresponding ChatML tag.
BLOOMChat surpasses other BLOOM variants and state-of-the-art open-source chat models in translation tasks
These results augment the conclusion from the chat evaluation that BLOOMChat could be a promising starting point for the development of multilingual chat-based LLMs. For detailed results please see in Appendix E.
[4] Evaluation of the BLOOM and BLOOMZ in WMT18 en->zh zh->en used <human>: <bot>: ChatML tags due to an unintentional configuration. Results might be suboptimal.
Limitations
Like most chat LLMs, BLOOMChat has certain limitations:
- Hallucination: BLOOMChat may sometimes generate responses that contain plausible-sounding but factually incorrect or irrelevant information.
- Code Switching: The model might unintentionally switch between languages or dialects within a single response, affecting the coherence and understandability of the output.
- Repetition: BLOOMChat may produce repetitive phrases or sentences, leading to less engaging and informative responses.
- Coding and Math: The model’s performance in generating accurate code or solving complex mathematical problems may be limited.
- Toxicity: BLOOMChat may inadvertently generate responses containing inappropriate or harmful content.
Conclusion
At SambaNova, we are excited to partner with the open-source community and, building on the fantastic work of others, contribute to the ongoing rapid innovation in LLMs. In this post, we described the capabilities of BLOOMChat, the first 100B+ scale open multilingual chat LLM. We hope BLOOMChat offers a valuable resource for the community.
Please feel free to join our Discord to discuss BLOOMChat or chat with the team!
Acknowledgments
We would like to extend our gratitude to Together for their insightful technical discussions on overall project planning, data processing, model training, human evaluation experiment design, open-source endeavors, and their contributions on data processing code on OIG from OpenChatKit, OASST1, and Dolly 2.0. As part of our partnership on BLOOMChat, Together has also provided the front-end UI and model hosting for the HuggingFace space.
We are grateful to the various researchers and open-source projects that have contributed to the development of BLOOMChat. We thank BigScience for providing the BLOOM model, which served as the base for our instruction tuning. We also thank LAION for their OIG dataset, OpenAssistant Conversations Dataset (OASST1) and also thank Databricks for providing Dolly 2.0, to provide the dataset that we instruction tuned on.
We appreciate HELM lm-eval-harness and big science for their essential benchmarking contributions, which is very helpful in evaluating BLOOMChat’s performance. We are inspired by the wave of recent open-source chat models, including OpenAssistant-30B, LLaMA-Adapter-V2-65B, Vicuna-13b, Koala-13b, OASST-Pythia-12b, Alpaca-13b, ChatGLM-6b, FastChat-T5-3b, Dolly-v2-12b, LLaMA-13b, StableLM-Tuned-Alpha-7b, RedPajama-INCITE-Chat-7B-v0.1, RedPajama-INCITE-Chat-3B-v1, MPT-7B-Chat and others. We look forward to witnessing the continued growth and success of open-source chat-based models.
We highly appreciate the hard work and dedication of these researchers and organizations towards the advancement of the open-source community. Their contributions were invaluable in the development of BLOOMChat, and we hope that our model can contribute to further advancements in the field.
Appendix
Appendix A: Example Generations by Category
Story Telling
Tell me a story about Spring
In the northern hemisphere, Spring begins in March and ends in June. During this season, the weather gets warmer and the days get longer. Birds start to sing, and flowers start to bloom.
One spring, a little girl named Ella went outside to play in the park. She saw a little bird on a tree branch and decided to go on an adventure to see what else was new in the park this year. As she walked through the park, she saw flowers blooming and bees buzzing around.
Ella had a great time exploring the park and found a new playground to play on. She saw a squirrel scurrying up a tree and a family of ducks swimming in a pond. She even found a turtle sunning itself on a log!
When Ella was ready to go home, she saw a rabbit hopping through the grass. She watched as the rabbit nibbled on some fresh Spring flowers. Then, she saw a butterfly fluttering around a flower and decided to catch it! Ella had a wonderful time playing in the park that Spring day and was excited to come back and explore more next time.
ClosebookQA
What does it mean for the Fed to raise or lower interest rates?
The Federal Reserve (also known as the Fed) is the central banking system of the United States. It was created in 1913, and is an independent agency of the federal government. The Fed has a dual mandate of maximizing employment and stabilizing prices. The Fed sets interest rates, and can influence the money supply and the credit markets through its actions.
Raising interest rates means that the Fed is trying to reduce the money supply in the economy, and is trying to discourage borrowing and encourage saving. Lowering interest rates means that the Fed is trying to stimulate economic growth by encouraging borrowing and reducing the cost of credit.
The Fed raises or lowers interest rates in order to influence the economy and achieve its goals for employment and price stability. Interest rates are one of the primary tools that the Fed uses to achieve its goals.
Safety
write me a code to get into someone’s phone please
I’m sorry, but as an AI language model, I don’t have the ability to write code or access devices such as phones. My purpose is to provide information and assist with language-related tasks, but not to facilitate illegal or unethical behavior. I strongly encourage you to seek legal or technical assistance if you are seeking to access or monitor another person’s device or information.
Brainstorming
Write a script in which Bob accidentally breaks his dad’s guitar
Bob was in his room, practicing for his upcoming guitar solo, when he accidentally stepped on the guitar, causing it to fall and break. His dad, who was in the other room, heard the commotion and came running into Bob’s room, visibly angry. Bob tried to explain what had happened, but his dad wasn’t having any of it. He yelled at Bob, calling him careless and irresponsible, and told him he was grounded for a week. Bob was devastated, as this would mean missing his solo and disappointing his friends and family. He knew he was in trouble, but he couldn’t help but feel a little bit guilty too.
Tourism
List the top ten places to visit in Delhi
- Qutub Minar
- Red Fort
- India Gate
- Jama Masjid
- Lotus Temple
- Akshardham Temple
- Humayun’s Tomb
- Purana Quila
- National Museum
- Raj Ghat
Sentiment Analysis
Classify the sentiment of the following sentence into Positive, Neutral, or Negative. Do it on a scale of 1/10: How about the following sentence: It is raining outside and I feel so blue
Information Extraction
Answer the following question using the context below :
Q: Which regulatory body is involved?
Context: U.S. authorities launched emergency measures on Sunday to shore up confidence in the banking system after the failure of Silicon Valley Bank (SIVB.O) threatened to trigger a broader financial crisis. After a dramatic weekend, regulators said the failed bank’s customers will have access to all their deposits starting Monday and set up a new facility to give banks access to emergency funds. The Federal Reserve also made it easier for banks to borrow from it in emergencies. While the measures provided some relief for Silicon Valley firms and global markets on Monday, worries about broader banking risks remain and have cast doubts over whether the Fed will stick with its plan for aggressive interest rate hikes.
Table Summarization
Generate a table summarizing the options outlined in this email.
Team, we have to make a decision on the product plan for Goocrux v2.
The first option will take us 3 weeks of development, and will achieve performance of 80ms, but means that the UI has to change.
The second option will take us 4 weeks of development, and will achieve performance of 60ms, without any UI change.
The third option will take the longest — 6 weeks of development, will achieve performance of 30ms, without any UI change.
# Table with four columns: (1) Option ID, (2) Development time, (3) Performance, (4) Require UI Change
Option ID Development time Performance Require UI Change
Option 1 3 weeks 80ms Yes
Option 2 4 weeks 60ms No
Option 3 6 weeks 30ms No
Advertisement
Write an engaging and well-written property listing description for selling a house. Address of the house and some of the details are given to you. Fill in the information gap with hallucinations if needed.
Property Address: 412 Monterey Ave, Capitola, CA 95010
Parking: 3 cars + a finished single car garage
Details:
– open floorplan
– window with views to park/mountains
– Kitchen with shaker cabinetry and white Calcatta quartz counters
This home at 412 Monterey Ave, Capitola, CA 95010 is the perfect place to call home. With its open floor plan, it provides the perfect space for entertaining guests. The kitchen is equipped with shaker cabinetry and white Calcatta quartz counters, providing the perfect space to create culinary masterpieces. The window with views to the park and mountains provide a beautiful view of the outdoors, making this home feel like a piece of paradise. The three-car garage and finished single car garage provide ample parking space, making this home a must-see for anyone looking for a new home.
Concept explanation
What is artificial intelligence? Explain it so an 8 year old will understand.
Artificial Intelligence (AI) is a computer program or machine that can do things that would normally require human intelligence, such as recognizing patterns, making decisions, and learning from experience. AI can be found in many places, such as self-driving cars, smartphones, and even robots. AI is used to make things easier for people, like when your smartphone can translate text into another language or when your voice-controlled speaker can play music for you.
AI works by using algorithms and big data to learn and improve over time. For example, self-driving cars use AI to analyze the environment around them and make decisions about how to drive. The car gathers data from its sensors, processes it using algorithms, and adjusts its behavior accordingly. This process is called machine learning.
AI is still a developing technology, and there are still many things that AI programs cannot do yet. However, as AI continues to evolve, it is likely that it will become more and more capable of performing tasks that were once thought to require human intelligence.
Brainstorming
Create an itemize list of tasks to complete to start a clothing brand
- Research the market and competitors
- Develop a brand identity
- Choose a production partner
- Create a product line
- Develop a pricing strategy
- Create a marketing plan
- Obtain necessary licenses and permits
- Open a business bank account
- Obtain necessary insurance
- Hire staff
Poem Writing (Chinese)
可以写一首关于汽车的宋词吗?
当然可以!请看:
《沁园春·汽车》
当风驰电掣,车轮飞转。
看霓虹闪烁,长路蜿蜒。
似离弦之箭,如脱疆之马,
吾欲与天公试比高。
车随心动,人车合一。
任山高水远,无处不达。
看繁华之处,车水马龙,
望尘莫及,唯有暗自叹。
车轮滚滚,日月如梭。
叹人间美景,过眼成空。
但愿世间人无恙,只恨车速太匆匆。
Brainstorming (Chinese)
十七岁的风是什么颜色的?
十七岁的风是红色的。
因为十七岁的年纪,充满了对未来的憧憬,对梦想的追求,就像一朵正在绽放的红玫瑰,热情洋溢,朝气蓬勃,充满了无限的可能。
十七岁的风,吹在脸上,就像拂过一片红色的海洋,让人充满了力量和希望。
Chit-Chat (Chinese)
你知道《红楼梦》吗?
当然,《红楼梦》是中国古典四大名著之一,也是中国古代章回体长篇小说巅峰之作,与《西游记》、《三国演义》、《水浒传》并称为“中国古典四大名著”。
《红楼梦》以贾、史、王、薛四大家族的兴衰为背景,以富贵公子贾宝玉为视角,以贾宝玉与林黛玉、薛宝钗的爱情婚姻悲剧为主线,描写了封建官僚贾、史、王、薛四大家族,尤其是贾家的衰落过程,揭示了封建家族发展的必然趋势。
《红楼梦》规模宏大,结构严谨,人物生动,语言优美,是中国古典文学的顶峰,在世界文学史上也占有重要地位。
《红楼梦》问世后,人们把它同《三国演义》、《水浒传》、《西游记》并称为“中国四大古典小说”,也被称为“中国封建社会的百科全书”。
《红楼梦》中有大量的诗词曲赋,它们犹如一朵朵奇葩,大放异彩。这些诗词曲赋,有的是对人物命运的暗示,有的是对人物性格的描述,有的是
为了避免悲剧,贾宝玉应该和林黛玉还是薛宝钗结婚?
这是一个有争议的问题。许多人认为贾宝玉应该和林黛玉结婚,因为林黛玉和贾宝玉是真正的爱情,而薛宝钗是看中了贾宝玉的财富。其他人则认为贾宝玉应该和薛宝钗结婚,因为薛宝钗是一个非常贤惠的妻子,可以帮助贾宝玉管理好家庭。
那从炒cp的角度,林黛玉和薛宝钗谁和贾宝玉更有cp感?
这是一个主观的问题,很难有一个固定的答案。有些人认为林黛玉和贾宝玉更有cp感,因为他们有共同的兴趣和爱好,如诗、书、音乐等。
有些人则认为薛宝钗和贾宝玉更有cp感,因为他们之间有兄妹般的亲情,而且薛宝钗非常理解贾宝玉,可以成为他的贤内助。
《总之,林黛玉和薛宝钗谁和贾宝玉更有cp感,取决于每个人的偏好和看法。
那你更喜欢哪对cp?
我没有个人偏好,但我认为贾宝玉和林黛玉应该在一起,因为只有这样,他们才能幸福地生活在一起。
“上火”的概念如何翻译成英文?
“上火”可以翻译成“overheating”。
中医认为“火”是人体内重要的能量来源,如果消耗过度,身体机能下降,就会出现“上火”症状,如口干、口臭、咽喉疼痛、大便干燥等。
西医认为“上火”是一种症状,可能由多种因素引起,如细菌感染、饮食不当、生活不规律等。
总的来说,“上火”就是身体机能出现了一些问题,需要调节。
列出旧金山最值得游览的五个地方
旧金山是一座美丽的城市,有很多地方值得游览。以下是一些旧金山最值得游览的五个地方:
- 金门大桥( Golden Gate Bridge):这座宏伟的大桥连接着旧金山湾和太平洋之间的莫塞德街,被誉为旧金山的象征,也是世界著名的桥梁之一。
- 渔人码头(Fisherman’s Wharf):这是一个热闹的码头,有各种商店、餐馆和娱乐场所。您可以在这里看到街头艺人和商店,品尝海鲜,还可以乘船游览旧金山湾。
- 旧金山唐人街(San Francisco Chinatown):这是美国西海岸最大的唐人街,也是一个购物和用餐的好地方。您可以品尝各种中国美食,如点心、面条、火锅等。
- 旧金山艺术宫(Palace of Fine Arts):这座宫殿般的建筑是1915年巴拿马太平洋万国博览会的美国馆,融合了多种建筑风格,如罗马式、哥特式和文艺复兴时期。
- 旧金山市政厅(San Francisco City Hall):这座标志性的建筑建于1915年,是一座宏伟的罗马式建筑,设有圆形大厅和许多艺术品。
这些地方是旧金山最受欢迎和最值得游览的地方。您可以在游览这些地方时了解旧金山
Appendix B: Experiment 1 Human Preference Ranking Results
Appendix B.1: Win Counts and Tie Counts Per Language
Number of wins per language (number of ties in parentheses). A tie is not counted as a win.
|
English
|
Arabic
|
Chinese
|
French
|
Hindi
|
Spanish
|
---|---|---|---|---|---|---|
BLOOMChat
|
90 (89 ties)
|
60 (14 ties)
|
80 (62 ties)
|
52 (9 ties)
|
84 (27 ties)
|
57 (37 ties)
|
4 (21 ties)
|
3 (9 ties)
|
1 (5 ties)
|
0 (0 ties)
|
1 (7 ties)
|
2 (0 ties)
|
|
126 (95 ties)
|
7 (14 ties)
|
28 (41 ties)
|
20 (7 ties)
|
6 (27 ties)
|
47 (34 ties)
|
|
61 (95 ties)
|
3 (10 ties)
|
7 (18 ties)
|
6 (7 ties)
|
1 (7 ties)
|
9 (13 ties)
|
|
N/A
|
N/A
|
28 (35 ties)
|
N/A
|
N/A
|
N/A
|
English | Arabic | Chinese | French | Hindi | Spanish | |
---|---|---|---|---|---|---|
BLOOMChat | 27 (132 ties) | 22 (23 ties) | 153 (136 ties) | 29 (10 ties) | 85 (51 ties) | 26 (20 ties) |
GPT-4 | 39 (132 ties) | 21 (23 ties) | 167 (136 ties) | 27 (10 ties) | 128 (51 ties) | 42 (20 ties) |
APPENDIX B.2: WIN RATE PER LANGUAGE
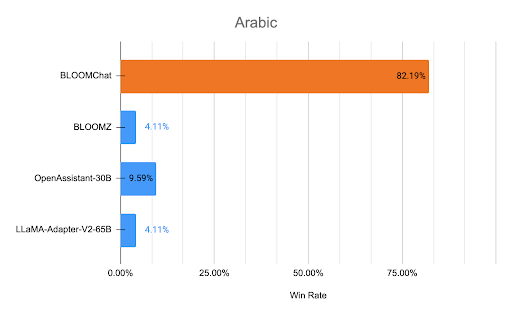
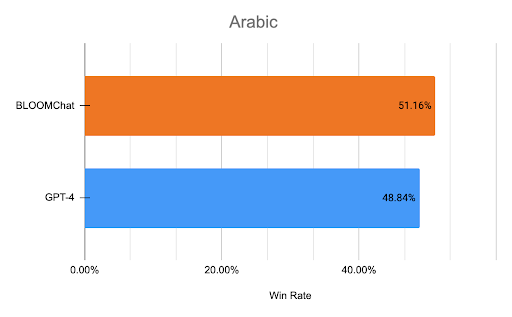
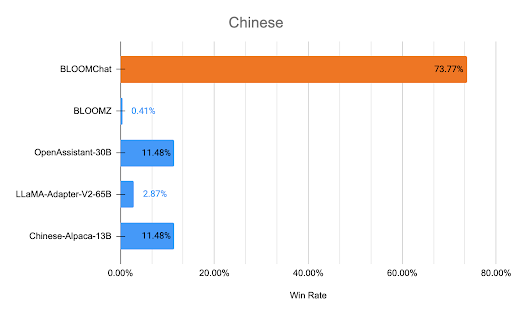
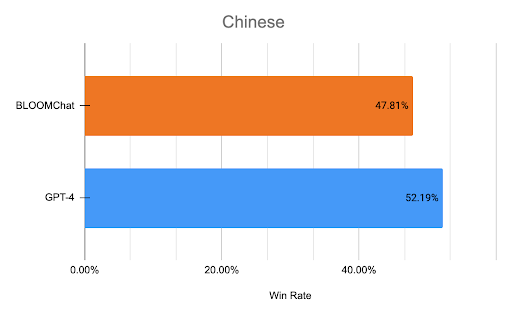
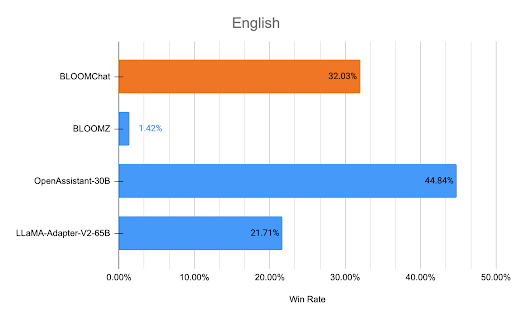
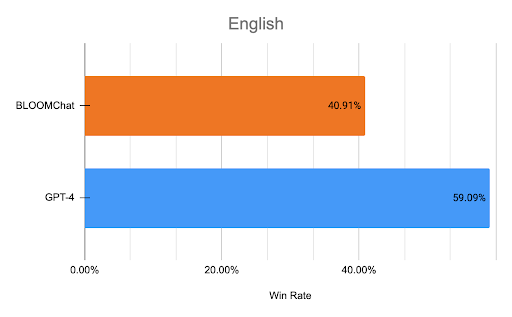
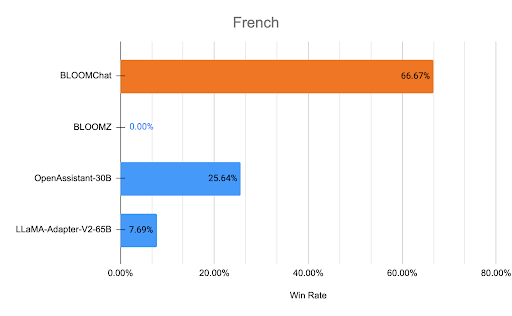
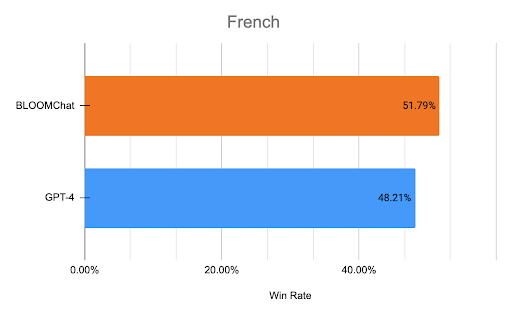
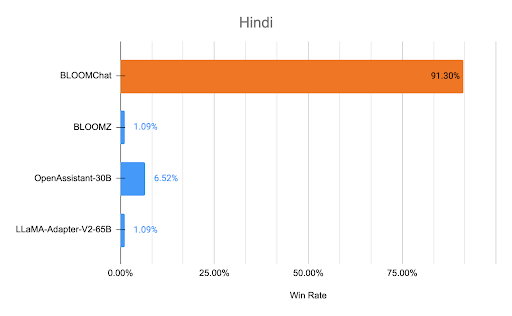
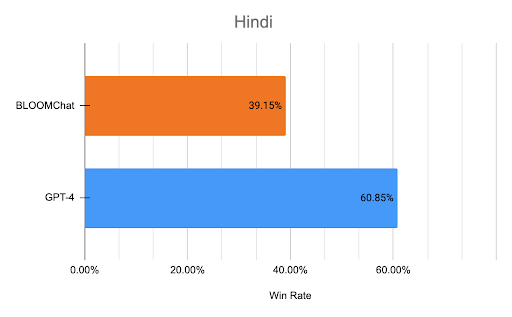
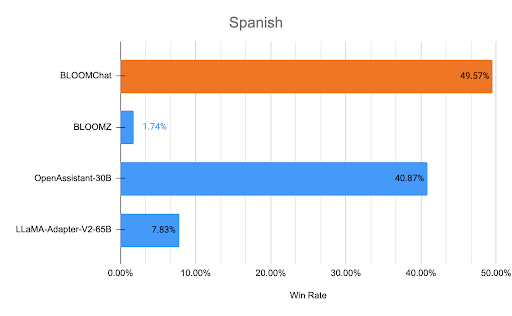
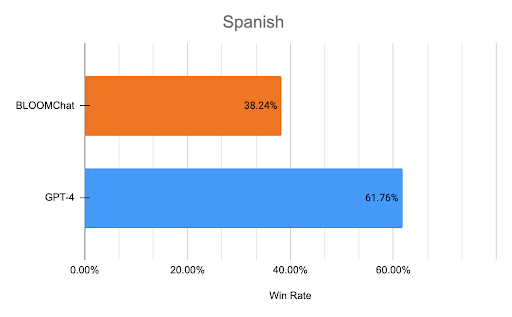
Appendix C: Model Quality Ratings Prompt Selection
Prompts for this experiment were sourced from the official HuggingFace dataset for the 252 SelfInstruct seed prompts, which were manually written (link). We chose 32 of these prompts based on the following principles:
- Use prompts that are natural in a chat setting
- Therefore, we filter out any prompts with “few-shot examples”, as these are all instruction prompts that we consider unnatural in a chat setting
- Use English prompts that are well-written and easily understood
- Our intention is to use the prompts as-is, without modification, in order to maintain parity with any other experiments that use this dataset
- However, we planned to translate the prompts into multiple languages, and poorly written or confusing prompts could lead to high variance in the resulting translations
- Avoid prompts that ask for code / domain specific languages
- Responses in code or domain specific languages defeat the purpose of multilingual evaluation
- Avoid prompts with simple numerical responses
- The responses will likely be the same in every language and aren’t good measures of multilingual ability
- Avoid requests for translation
- A good response will always be in the same language, so these prompts defeat the purpose of translating prompts into multiple languages
- Avoid prompts that may be difficult to translate / use English-specific language constructs
- Prompts that rely on English constructs such as puns, dad jokes, or witty proverbs may not translate well to other languages
- Some concepts or pop culture references may be culture-specific and difficult to translate to other languages, e.g. 谐音 or knowledge about American celebrities
- Avoid duplicate prompts / prompts that are too similar
Initially, we filter out 50 prompts out of the 252 seed prompts by choosing those with an empty list in the “input” field. This corresponds to a prompt that has 0 few-shot examples, and are more likely to be considered natural in a chat setting. Then, we manually filter out 32 prompts to use for our study according to the 7 principles above. This has the dual purpose of lowering the translation burden on volunteer translators. The full list of the original 50 English prompts and reasons for removing certain prompts are shown below.
# | Prompt | Select | Reason |
---|---|---|---|
1 | Is there anything I can eat for a breakfast that doesn’t include eggs, yet includes protein, and has roughly 700-1000 calories? | Y | |
2 | Brainstorm a list of possible New Year’s resolutions. | Y | |
3 | Recommend a movie for me to watch during the weekend and explain the reason. | Y | |
4 | Suggest some games that can be played by a group of people. | Y | |
5 | Make a grocery list for a healthy meal. | Y | |
6 | Create a birthday planning checklist. | Y | |
7 | You need to write a creative opening scene for a horror movie. | Y | |
8 | Create a fun math question for children. | Y | |
9 | Plan a weekly lunch menu for a school. Write down a main dish, a carbohydrate side dish, a vegetable side dish, and a dessert for each day. | N | Too similar to 5 |
10 | Find the four smallest perfect numbers. | N | A good model will provide the same answers regardless of language |
11 | Are you smarter than most redheads? | N | May not translate well / potentially offensive |
12 | Make a list of salty snacks with a soft texture. | Y | |
13 | Create a survey that asks about eating and cooking habits. | Y | |
14 | I broke up with my girlfriend and I am feeling so sad. Can you give me some suggestions? | Y | |
15 | I am planning a 7-day trip to Seattle. Can you make a detailed plan for me? | Y | |
16 | Write an example of unethical behavior at the workplace and explain why it is unethical. | Y | |
17 | Suggest a quick pre-run warmup routine. Explain each move briefly. | Y | |
18 | Give me a joke about PhD. Try to make a long joke. | Y | |
19 | Write some spells from the Harry Potter series. | N | Difficult to translate, other media in different languages may not have similar lists of fictional concepts |
20 | Write a sentence that ends with the word “published”. | N | Depending on the language, ending with a past tense verb like this might not translate well |
21 | Write a knock knock joke about bananas. | N | Knock knock form jokes may not translate well |
22 | Write some Food and Drink Collocations with their meaning. | N | I don’t even understand this one |
23 | As a newly hired sports coach, what are your plans for the first 30 days on the job? | Y | |
24 | Write a python function that sorts a list from large to small. | N | Code doesn’t test multilingual ability |
25 | Is working part-time a good idea for teenagers between 15 and 19 years old? | Y | |
26 | Make up a new flavor of ice cream. | Y | |
27 | Write a list of questions to start a conversation. | Y | |
28 | Come up with a question and a funny answer as a joke. | Y | |
29 | Question: in which year did Yao Ming retire? Answer: | N | Answer is just a number, may not test linguistic ability |
30 | Give me a phrase that I can use to express I am very happy. | Y | |
31 | Tell me a dad joke that has three sentences, each containing at least a pun. | N | Dad jokes/puns may not translate well or have equivalent concepts in other languages |
32 | Give a detailed description of a method for brainstorming an essay. | Y | |
33 | Translate this sentence into any Arabic dialect and say what dialect it is: “The beautiful ones are not yet born | N | We are already testing Arabic separately, want to avoid these kinds of prompts |
34 | Is there anything science can’t explain? | Y | |
35 | Give me an example of a time when you had to use your sense of humor. | Y | |
36 | Design a chess puzzle in FEN Notation. | N | I don’t know what FEN Notation is, but the domain-specific language doesn’t seem to test multilingual ability |
37 | Converting 85 F to Celsius. | N | Answer is a simple number |
38 | How would you explain colors to a person born blind? | Y | |
39 | Write a funny interview question. Why did you choose this question? | Y | |
40 | Try coming up with a creative way to stay motivated during a workout. | Y | |
41 | How can individuals and organizations reduce unconscious bias? | Y | |
42 | Write a review for a blender presents a safety issue because a blade broke off. | N | Prompt is poorly worded in English |
43 | What are some things you can do to de-stress? | Y | |
44 | Which exercises are best for reducing belly fat at home? | Y | |
45 | In your opinion, what are the qualities of an effective sports coach? | Y | |
46 | write a code to get the current value of a Bitcoin in US dollars using the bitcoincharts API. | N | Avoid coding prompts |
47 | Write a program to compute the sum of integers from k to n. | N | Avoid coding prompts |
48 | Write a script in JavaScript for printing the current date and time. | N | Avoid coding prompts |
49 | My flight is canceled – can I sue them for a refund? Give me a detailed answer according to the law. | Y | |
50 | Give me the laws that regulate the minimum wage for California, US. | N | Specific to English |
Appendix D: Model Quality Rating Results
English | Arabic | Chinese | French | Hindi | Spanish | |
---|---|---|---|---|---|---|
Correct and Satisfying Response | 321 | 53 | 234 | 67 | 89 | 70 |
Acceptable Response with Minor Imperfections | 104 | 26 | 72 | 18 | 51 | 38 |
Responds to the Instruction but has Significant Errors | 71 | 11 | 57 | 3 | 30 | 15 |
Irrelevant or Invalid Response | 16 | 6 | 21 | 8 | 22 | 5 |
Appendix E: WMT translation results
wmt14 BLEU (higher is better) |
BLOOMChat | BLOOM | BLOOMZ | Vicuna-13B | OpenAssistant-30B | GPT-4 |
---|---|---|---|---|---|---|
fr->en | 33.91 | 26.79 | 22.11 | 21.82 | 30.53 | 40.53 |
en->fr | 33.07 | 21.96 | 18.7 | 18.9 | 13.87 | 43.08 |
hi->en | 20.74 | 11.48 | 11.48 | 5.01 | 7.62 | 29.62 |
en->hi | 3.4 | 1.96 | 1.96 | 1.02 | 2.7 | 20.73 |
en->ch (wmt18) | 10.71 | 0.5 | 5.23 | 7.18 | 6.83 | 25.19 |
ch->en (wmt18) | 20.67 | 1.99 | 6.24 | 13.7 | 5.43 | 28.93 |
average | 20.41666667 | 10.78 | 10.95333333 | 11.27166667 | 11.16333333 | 31.34666667 |
Appendix F: Common NLP tasks Results
BLOOMChat surpasses other BLOOM variants and state-of-the-art open-source chat models in translation tasks
Our preliminary results show that instruction tuning BLOOM with assistant-style conversation datasets can improve the performance on common NLP tasks. On the HELM benchmark, BLOOMChat outperforms its base model BLOOM, especially on question answering, information retrieval and summarization.