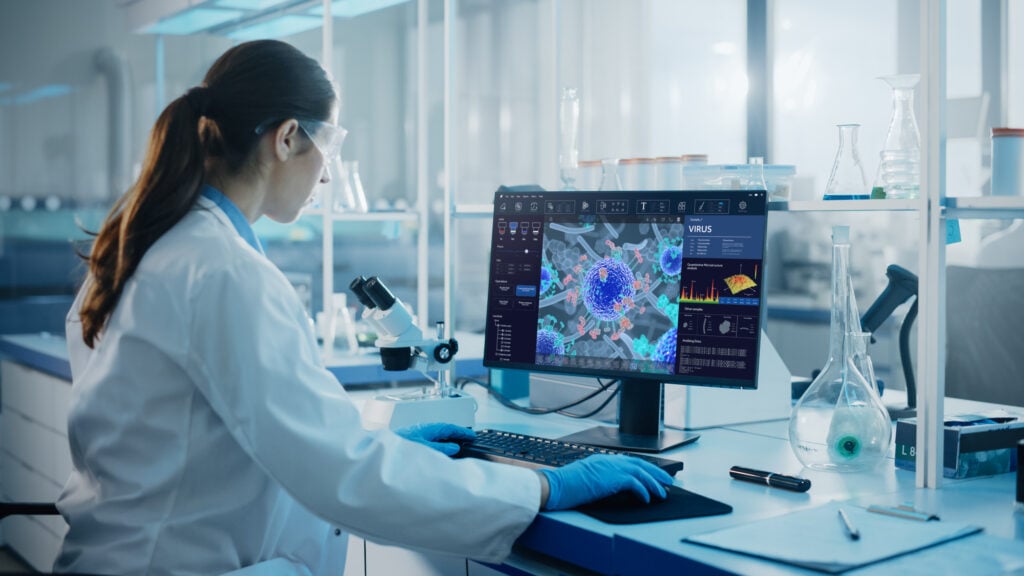
In September 2022, SambaNova unveiled its new DataScale® SN30 system, delivering new innovations at every layer of the AI stack. Our philosophy for this release was simple: the world is entering a new era of AI powered by foundation models, and leading organizations need a new AI platform to capture the powerful benefits foundation models can deliver.
One of the areas where SambaNova sees the biggest application for foundation models and deep learning is accelerating scientific research and discovery across domains like advanced medical research, complex multi-physics simulations, and large scale seismic analysis.
In fact, these topics and more will be the focus of our conversations with customer and industry leaders at this week’s SC22 conference. The SC conference is always an exciting time for SambaNova because the scientific research community has always been a core part of our customer base and a focus of our product innovation.
Today, I wanted to share several exciting announcements from SambaNova as part of our presence at the SC22 conference, including a significant expansion of our long time collaborations with leading research organizations, such as Argonne National Labs, a revolution in what is possible for computer vision, and the potential for foundation models to power a new era of scientific discovery.
5123 resolution and beyond – a new paradigm in computer vision
For computer vision use cases and applications that analyze 3D data, image resolution has a significant impact on accuracy and results. In fact, in several recent experiments, simply increasing image resolution from 1283 to 5123 delivered a sizable increase in accuracy without any significant post-processing optimizations.
To demonstrate what I mean, let’s look at two different images that have been generated with a computer vision model: the first using 1283 resolution and the second using 5123 resolution. Both these images are predictions from the same dataset, in this case seismic data which is used to predict the location of oil and gas deposits.
Ground Truth |
1283 Image |
5123 Image |
As you can see in the images above, the 1283 resolution image is not only less accurate in terms of model evaluation metrics, but it also ‘qualitatively’ less accurate compared to the ‘ground truth’ version of the image. Meanwhile, the 5123 image is extremely accurate and closely resembles the ‘ground truth’ version of the image. This qualitative accuracy improvement is particularly important to seismic data analysts, who use these images as a core part of the process of identifying resource deposits, which are potentially worth billions of dollars.
Higher resolution is beneficial to more than just seismic analysis: there are a number of different domains that can benefit from the accuracy gains resulting from an increase in image resolution:
- Medical imaging: Analyzing high resolution 3D medical images to predict the presence and location of tumors, helping doctors accelerate treatment and save lives.
- Particle Physics: Analyzing images to determine the presence of subatomic particles in testing environments.
Large Language Models and Transformer Models for Science
Large Language Models (LLMs) have been one of the most exciting areas of AI innovation over the past several years. LLMs are an emerging type of transformer model that can deliver a high degree of accuracy across dozens of different tasks. LLMs are able to achieve this versatility by not just processing language data, but by developing an understanding of language structure and context. However, this approach to understanding the structure and context of data isn’t limited to just language data – it can be applied to multiple different scientific domains. There are many areas of research that can benefit from this application of this methodology. SambaNova’s world record performance for large language models is enabling leading research organizations to perform more experiments and make discoveries faster. Here are a few of the most exciting use cases where transformer models are accelerating scientific research and discoveries:
- Drug discovery: Learning molecular fingerprints and chemistry-relevant representations to accelerate drug discovery.
- Covid-19 research: Using transformers to study and analyze Covid-19.
- Chemical synthesis: Transformers can understand strings of chemical bonds for materials research and other applications.
- Computer-aided design: Developing an understanding of design structure to improve design output and automate some design tasks.
Deep Learning Accelerated Surrogate Models
Surrogate models are deep learning models that replace one or more components of larger multi-physics simulation workloads. By optimizing specific parts of the simulation using deep learning, research organizations can greatly accelerate the overall performance of these simulations, enabling more and faster experiments. However, from a technical perspective, the GPU/CPU-based architectures used to compute simulation workloads struggle to deliver performance on the sparse, detailed deep learning surrogate models. Research organizations must either operate both types of workloads on the same architecture, or tradeoff gained speed with added latency from shifting data between different systems optimized for each of the different workloads. The SambaNova platform overcomes this challenge by significantly improving the performance of sparse, detailed deep learning models compared to GPU-based architectures, even taking into consideration the additional latency requirements of two different systems. Examples of areas where surrogate models can be used include:
- Weather forecasting: Applying deep learning to analyze publicly available weather and climate data to improve forecasting accuracy.
- Computational fluid dynamics: Utilizing surrogate models to improve predictions of flow dynamics such as velocity, density, pressure, and temperature in applications such as engine research.
You can read more about several announcements we made on these areas research here:
- SambaNova delivers next-generation DataScale system to Argonne National Laboratory
- SambaNova accelerates seismic analysis, enabling faster resource discovery for oil and gas companies
We look forward to continuing to expand our collaboration with the research, scientific, and supercomputing community in these exciting areas and more. Join us at booth #3042 at SC22 in Dallas. We look forward to seeing you there.